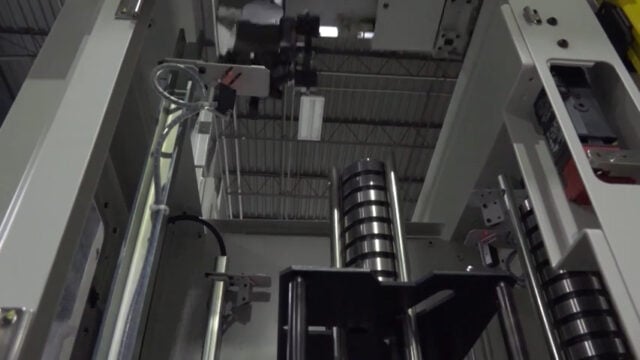
The latest news and machining technologies from North America.
Are you looking to promote your business and products? Click here
Featured
Are you looking to promote your business and products? Click here
Featured
Open houses, exhibitions, seminars, grand openings…all events can be found on our events page, here are some of the highlights.
1 – 3 April 2025 | PMTS 2025 | Cleveland
Find out more8 – 10 April 2025 | Rapid + TCT 2025 | Detroit
Find out more12 – 15 May 2025 | Automate 2025 | Detroit
Find out more13 – 15 May 2025 | Manufacturing Technology Series EAST | Massachusetts
Find out moreClear your schedule and grab your favorite cafecito because Episode 178 of The Gunn Show is…
The latest episode of The Gunn Show is a full-throttle ride into the world of gear…
Founded in 1995, Paramount Machine has grown exponentially, relocating four times to accommodate its expanding operations.…
If you’ve ever wondered what happens when a former Marine, an avid cyclist, and a manufacturing…
If you’ve ever wondered what happens when you combine a master of precision machining with a…
Jack Russell’s foray into manufacturing began with a bold move: investing his 401(k) to acquire Rolar…
Be first to see all the updates from MTDCNC
Our newsletters frequency varies dependant on content
All the latest deals from the industry feature on our newsletters